Dinosol: how to segment a supermarket's customer base
Problem
Identifying and quantifying the different customer groups of a supermarket is the first step in defining a differentiated CRM strategy for each customer group.
Solution
Development of two segmentation models (RFM and Clustering) to identify homogeneous groups and classify customers into them.
Results
We found groups of customers with different behavior and were able to describe them in terms of demographics, purchase pattern, life cycle, recency, frequency and value of purchase…
Context
No two customers are alike. Each has his or her own profile, preferences, shopping habits, basket of products, etc. How can we group customers with similar behavior? The large number of variables and volume of data in a supermarket’s transaction history makes segmentation not an easy challenge.
What are my objectives, what data do I need?
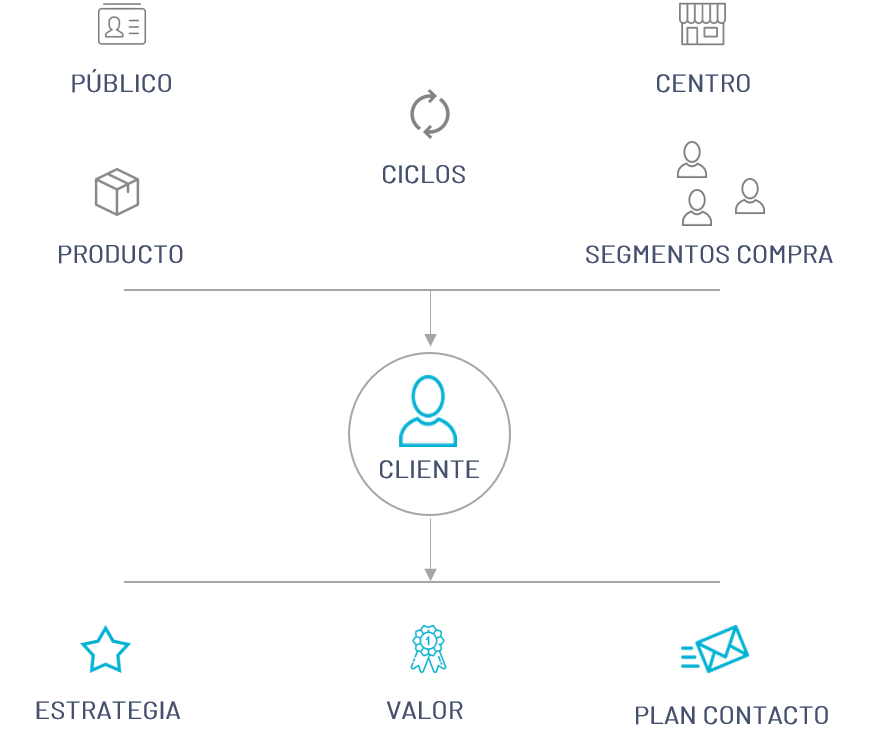
Defining the objectives leads us to ask what data I need and have available:
- Define the main strategy by group: recognition, up&cross selling, retention and abandonment.
- Define the investment volume and value proposition.
- Define the contact plan
Customer segmentation models...
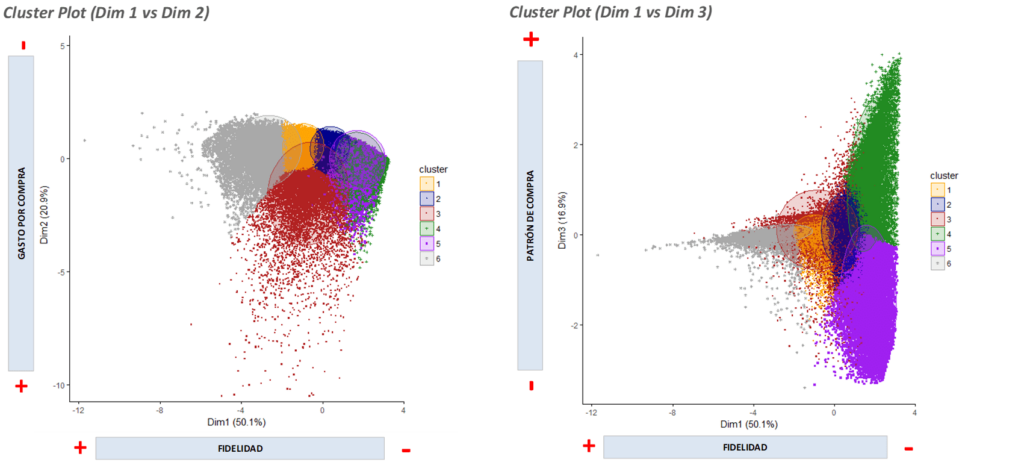
Developing a model is a process that goes through the following phases:
Exploratory Phase
- Summary statistics of variables by data type
- Distributions of variables by data type
- Correlations and significant associations between variables.
- Identification of relevant variables for demand estimation.
Preparation Phase
- Elimination of outliers
- Data transformation and creation of new variables: normalization, categorization, indexes, indicator variables, etc.
- Sample selection: Train (75%) and Test (25%).
Modeling Phase
Cluster model, in two stages. Exploration tool designed to discover the natural groupings of a data set. It allows the analysis of large databases through the construction of a cluster feature tree that summarizes the records. It allows to work jointly with mixed type variables (qualitative and quantitative).
Step1: Construction of the Cluster Features (CF) tree. Aggregation of cases, within the same node or forming another leaf node (high number of preclusters). We rely on the existing similarity, using distance measures.
Step 2: Application of the hierarchical method (Clustering of leaf nodes). We use an agglomerative clustering algorithm, producing a range of solutions. To determine the number of clusters, each solution is compared using Schwarz’s Bayesian Criterion (BIC) or Akaike Information Criterion (AIC).
Validation Phase
Comparison of the results of the random samples Train (75%) and Test (25%). The Cluster model obtained is correct if the results between the two samples are consistent with respect to the number of clusters and the profile of each cluster.
How to make the model actionable...
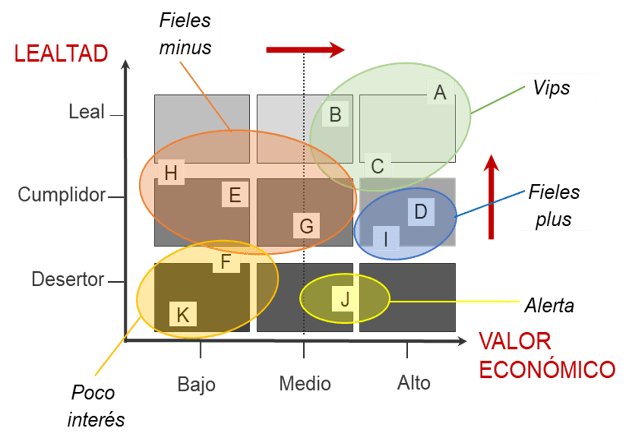
To make the results of the model more understandable, we describe and position each group obtained in a Loyalty / Value matrix. This graph helps us to perform a second grouping to be able to manage at the operational level the main strategies, the investment volume and the value proposition to each group, as well as the contact plan.